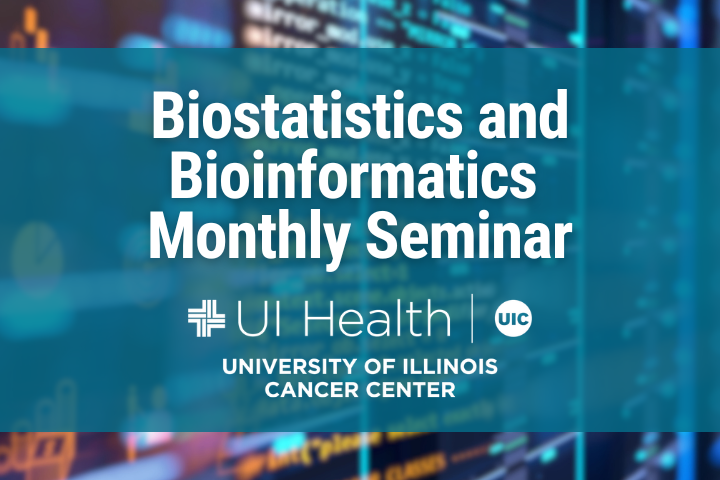
Biostatistics and Bioinformatics Monthly Seminar with Jie Chen, PhD
Analysis of High-Throughput Genomic Data: Modeling and Computational Perspective
Jie Chen, PhD
Professor of Biostatistics and Data Science
Department of Biostatistics, Data Science and Epidemiology (BDSE)
Associate Dean for Academic and Student Affairs
School of Public Health, Augusta University
Meeting ID: 834 2878 7778
Passcode: Sy6FTVFi
Abstract: The high-throughput sequencing technology has provided new opportunities of discovery in biomedical research. While the sequencing data pre-processing techniques have been very well developed and widely accepted by the research community, modeling and analyzing high-dimensional genomic data still post computational challenges. In this talk, I will share two completed projects. The first one is a framework on modelling sequencing data of multiple subjects for genomic feature discovery. Taking into consideration of the correlated structure of the data, we use the framework of a fused Lasso latent feature model to solve the problem and further proposed a modified information criterion for the tuning parameter selection when searching for common features shared by multiple samples. The second one is on jointly analyzing multiple types of genomics data, along with prognostic information, available within and across different studies. It has been a challenging and common task in modern statistical research to use all types of data to infer disease-prone genetic information and to link those features to cancer survival. We modelled the genomic, prognostic and survival datasets under a framework of an accelerated failure time with frailty (AFTF) to infer patients’ survival time. Simulation results confirmed the good performance of the approach. The approach was applied to the analysis of multiple genomic datasets of Giloblastoma Multiforme (GBM), a lethal brain cancer, and interesting genomic features are identified, and biological interpretations are explored.
This group meets monthly from Noon – 1 p.m.